An innovative neural-network implementation
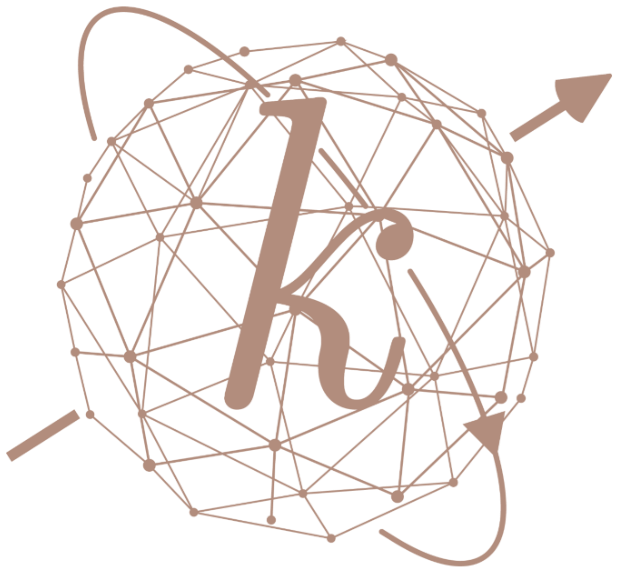
With k-NET, we envisage a neural-network implementation that is qualitatively different from all existing propositions. Instead of designing and structuring individual nonlinear elements (neurons) and their interconnections (synapses) in real space, we propose a different paradigm in which these elements are constructed in reciprocal or k-space of large dimensionality. We imagine to realize this concept of hyperconnectivity using populations of the eigen-excitations of ferromagnetic thin films – spin waves – as neurons. The key property of magnetization dynamics is its strong nonlinearity, which, when coupled with geometrical effects and external stimuli like applied fields and currents, translates into two useful features for neural networks: (i) nonlinear interactions through exchange and dipole-dipole interactions couple potentially all spin wave (SW) modes together, thereby creating high connectivity; (ii) the strength of the coupling depends on the population of each k mode, thereby allowing for synaptic weights to be modified dynamically. The breakthrough concept here is that real-space structuration is not necessary to achieve hyper-connectivity or reconfigurable synaptic weights.